Reflections on FMItech at Midwinter Conference 2021
February 9, 2021
by Casey Castellanos
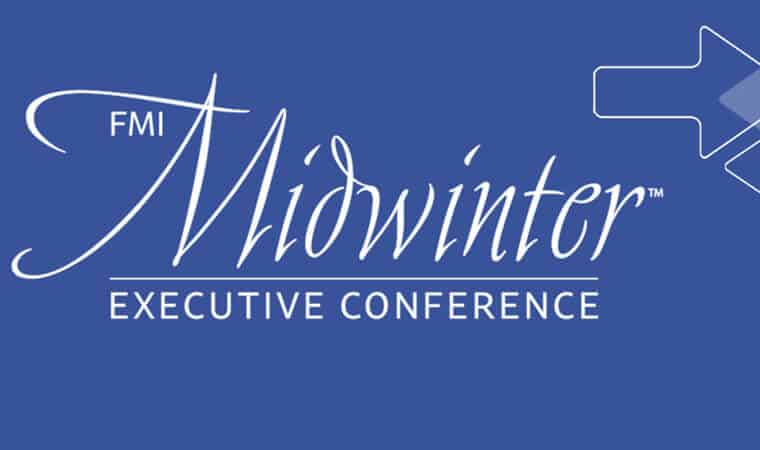
Over the past couple of weeks (Jan 25-29; Feb 1-4), we were at FMI Midwinter Executive Conference. We enjoyed talking with many retailers, wholesalers, product suppliers, and food manufacturers. We participated in an FMItech talk on January 26th. Sanish Mondkar, CEO and founder of Legion, spoke on the “Optimizing your Operational Performance” panel and was joined by Afresh Technologies, Planalytics, CB4, and Invatron.Â
Sanish discussed how AI has become the new superpower for leading grocers that use it to transform labor optimization and employee engagement. He shared how Legion WFM can help organizations decrease labor costs by 5-10% and dramatically increase employee engagement. Also, AI can automatically process customer demand, budgets, labor productivity standards, labor compliance regulations, employee skills, and employee preferences to create optimized labor plans that balance business needs with employee preferences.Â
The Q&A panel was lively, so we’ve excerpted it here to give you a look.
Q: We’ve heard about WFM capabilities today: demand signals, demand optimization, labor optimization, etc. Many of these features have been on the market for some time now. But I believe vendors are using new technologies like artificial intelligence (AI), machine learning (ML), and big data to create the next generation of WFM solutions. Do these technologies take WFM to the next level, and should retailers take a fresh look?Â
A: Yes, retailers should definitely take a look at the evolution of WFM. The evolutionary leap between legacy WFM and AI-powered WFM is dramatic. Using AI as a central “brain” for WFM can massively increase WFM process automation as well as drive better results using data. This approach enables automatic and continuous processing of enormous volumes of data, such as weather, school calendars, local events, sales, and transactions. And, it creates highly accurate demand forecasts, optimal labor guidance (that takes labor standards into account), business policies, and budgets. With the staffing plan in place, an AI-powered approach helps create schedules that are deeply personalized for each employee while still ensuring adherence to skills, compliance, and other scheduling policies. That extends not just to schedule creation but also ongoing schedule maintenance. Any employee requests and actions can be first serviced by the same central brain that governs initial schedule creation, resulting in high schedule efficacy while needing very low manager oversight. Legion’s customers have been able to cut the time their managers spend on scheduling in half by leveraging Legion’s AI-powered WFM platform.
Q: How does a retailer plan for the gap between launching these new solutions and the time it takes AI/ML to learn and build the models at that retailer. If each retailer has a different environment, how does that come into play here?Â
A: The way to solve that challenge is to use historical data to train the models. Discovering the patterns and nuances of the data over the past several months (or sometimes a couple of years) will give the models a lot more insight into how to operate going forward. If you apply this approach from day 1, you have the accuracy and the data insights you need to drive results. And then, when you apply this continuous learning to new data sets, you look for new patterns because more or less everything that’s happened before is already baked into the model. There’s no wait time, so retailers can derive the value from day one after the system goes live.
Q: Are we talking about each retailer having a slightly different model that’s developed, or is it the same model for each retailer, but some it’s tailored to that particular environment?
A: It’s not just each retailer, but even within the same retailer, it’s each location, and then within the same location and maybe each channel, there’s an omnichannel context that may have a different model. The platform picks the right model and the right attributes to drive that model. Legion WFM is built bottom-up, so it’s very precise and personalized to the retailer’s data set, location, and fleet. That’s what makes this solution so powerful because it’s an automated infrastructure that’s very personalized to every single data set. It’s able to drive the accuracy and results needed to improve operations.Â
Q: We’ve written a lot about new technologies and especially foundational technologies like AI/ML. There’s a notion that when these data models are developed and begin working by leveraging data from the retailer, the models produce better and better results – in a sense almost self-learning. Is that actually what’s happening? Does that mean retailers who implement these optimization solutions sooner than later will move up their productivity curve faster?
A: AI and Machine Learning (ML) are self-learning. As they work with retailer’s data, the models become increasingly accurate and respond automatically to changing business conditions. AI and ML automatically learn patterns from the past and apply them to the future. The models can accurately predict peak and lull periods at a granular level that was never possible before. As new “actual” data (e.g., new sales data, traffic, and more) is continuously fed to the models, new patterns are detected and automatically incorporated in the models. That enables the software to be self-learning at a very granular level of a dataset or a location, which is extremely powerful and nearly impossible to accomplish without machine learning.Â
The key to rapid success and moving up the productivity curve faster is to take the time at the beginning of the project to carefully consider the demand drivers for the business. Demand drivers are a measurable quantity that drives labor allocation, such as sales, item counts, number of transactions, and traffic. Ideally, at the start of a WFM project, the retailer provides historical data for these demand drivers to start the self-learning process. To make that easier for our customers, Legion offers an Advanced Data Science Adoption Service where our team of expert data scientists work with the customer to select their demand drivers and continuously optimize the AI/ML models to accelerate the productivity curve.
If you’d like to learn more and see how Legion WFM would work in your environment, request a demo.